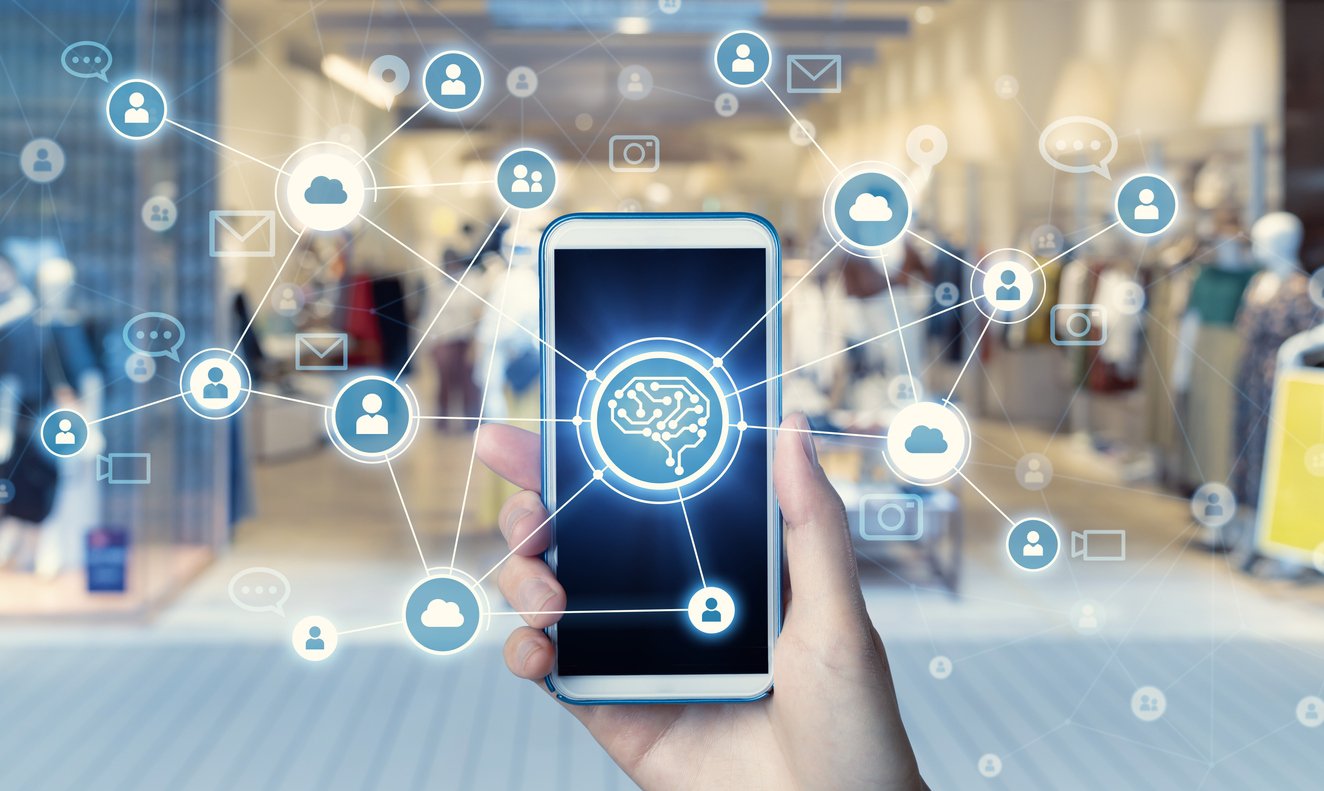
Who’d have thought that, one day, computers would be capable of recognizing and learning patterns to make decisions for themselves? The same goes for the e-commerce industry; nobody could have thought it would become what it is today. Machine learning has a lot of applications in the e-commerce industry that goes far beyond analytics. You can take the basic machine learning courses to get the idea of what it actually is.
For an industry heavily dependent on technological and digital advances, how is machine learning making its mark on the e-commerce industry? Let’s find out.
Better Search and Display
Machine learning can give users the ability to find exactly what they want based on their search query. At present, users find products on an e-commerce site using keywords, so the site owner must ensure that they have attributed those keywords to products that users are searching for.
Machine learning can enhance this by providing support for a broader set of synonyms. Smart machine learning looks for synonyms of keywords used, as well as similar phrases people use for the same query. Machine learning’s ability to do this comes from its capability to analyze a site and its metrics. This allows e-commerce sites to prioritize click rates and existing conversions while putting high-rated products on the top of the page. Machine learning can also accurately predict what customers want and then adapt product suggestions to best fit their needs. For example, you can set the search results to default sorting (“By Relevance” or “By Featured”) based on the search keywords as well as the customer’s profile and analysis. An e-commerce search engine using a customer’s profile has a higher probability of selling its listed products as the system predicts what the customer wants to see, rather than listing all products that match the keywords.
Chatbots
Chatbots backed by machine learning can provide a more “human” conversation with users by understanding structured data. Using machine learning, chatbots can be programmed with general information to respond to customer queries. The more the bot interacts with people, the more it will be able to understand an e-commerce site and its products/services. As more complex learning is employed, chatbots can do so much more, such as identifying potential upselling opportunities, delivering customized coupons by asking questions and addressing the customer’s long term needs. The cost of developing a custom chatbot for a site can range between $25,000 to $30,000, including design, development and integration. This can easily be financed using a small business loan.
While all of this is fair and good, one thing to always keep in mind is to respect your customer’s private information. A majority of chatbots are third-party applications and may be collecting or using your customer’s data to answer questions or create social media content automatically. Make agreements to lay down how much data can be used and make sure it’s anonymized.
More Ways to Make People Buy
Machine learning also creates opportunities for a host of virtual buying assistance with functionalities. This includes reminding users when prices drop below a specific limit and reminding them to get their air conditioner filter changed every few months. E-commerce sites can take this a step further by using coupons to reduce prices up to a limit, or for saving big, ahead of a holiday season.
Machine learning removes a lot of manual labor and guesswork required to identify consumer segments and allows e-commerce owners to send promotional content with differentiated pricing. Using profiles and analyzing browsing/purchase data, machine learning systems can create sales campaigns that meet multiple threshold criteria related to inventory, margins and repeated business.
Recommendation Engine
One of the biggest reasons why Amazon continues to dominate the e-commerce industry is because of its recommendation engine, which is based entirely on machine learning. Recommendation engines are like the rack of magazines or candy that you find at the checkout counter at a store. Their purpose is to remind customers about an item they may need, but have forgotten about. A recommendation engine learns and analyzes patterns from past user behavior from millions of data records. The engine will then be able to predict how a new user may browse the website, what they may be interested in and what people with similar profiles have behaved like in the past. When a user successfully makes a purchase, the engine sees if the recommendations were successful or not to further improve the algorithm.
Retargeting, Upselling and Discounts
Not every user visiting your site is going to end up buying something. Some may be just looking for product information, or some may actually add something to the cart, but back off at the end. Machine learning can help get more people to complete their purchase using dynamic retargeting, upselling and discounts. Machine learning enables e-commerce owners to better retarget users by looking at data to find out what had worked to convert similar profiles in the past through retargeting.
In some cases, a Facebook Ad may work; in other cases, a time-bound discount on the abandoned item may work. It all depends on what the data is pointing towards. Machine learning goes through millions of profiles, analyzing their behavior and outcomes to predict what will most likely work when trying to convert a customer.
Fraud Protection
The larger the amount of data, the easier it gets to catch any anomalies. Machine learning can identify patterns in data, identify what’s ‘normal’ behavior and notify admins when something is not ‘normal’. The most common application of this is in fraud detection. The problem of customers buying with stolen credit cards, or retracting payments after the item has been delivered, is rampant in e-commerce. Detecting and preventing such fraud is nearly impossible without machine learning that rapidly processes the repetitive data to detect frauds before they happen.
Better Inventory Management
One of the persistent problems in e-commerce is with inventory management. What’s astounding is that, even though $1 Trillion is tied up in capital, 46% of companies in the United States don’t track their inventory. Sellers sometimes oversell, shipments can take too long to deliver or forecasting must have been wrong. This affects the standard e-commerce motto of delivering the right products in the right place at the right quantity. Inventory management can be painstaking (especially for e-commerce sellers) if done manually – eventually impacting accurate sales predictions, which can subsequently lead to cash flow problems. Machine learning can make forecasting future demand a lot more precise. Not only will this help with easy supply chain management, but it also ensures that you understand your customers and their behaviors better.
Stock replenishment is necessary to avoid stock-outs and it ensures that customer demands are met in an accelerated timescale. Using machine learning algorithms, stock replenishment can be automated based on historical and current sales analysis. This can be configured for both “Pick up from store” and “Deliver to customer” arrangements.
Understand Your Customer Better
Customers demand more personalized experiences and, if you’re not ‘listening’ to them, they will find someone who will. The only way to meet this demand is by understanding their preferences better; it will be different for different types of customers. Since almost every interaction with the customer is digital, e-commerce sites collect huge amounts of customer data, including demographics, psychographics and behavioral data. The complex part is putting them all together and deriving actionable insights out of them for merchandising and marketing decisions. This is exactly where machine learning shines. Machine learning algorithms assess individual customer inventory and behavior data to predict exactly what they want. It can also deep dive into complex journey analytics and expose opportunities that you didn’t know even existed so that you can provide hyper-personalized experiences to customers.
Trend Analysis
Before putting products up for sale on an e-commerce site, you have to analyze their trend; is it a best-selling product, a normal seller or is it outdated? Trend analysis also has an impact on procurement, be it from an external vendor or internal fulfillment. Returned products will play a huge part in procurement if trends are not properly analyzed. Hence, it is critical to analyze the trend of a product, as it can reduce the overall size of the catalog, maintenance cost of products and improve space utilization at the warehouse. Using machine learning, this can be easily achieved as it analyses and cross compares product reviews, ratings and social media inputs. Products with low ratings can be discontinued from the site.
Most Useful Machine Learning Tools for E-Commerce
- Choice.AI helps create personalized e-commerce landing pages inclusive of smart banners in real-time.
- Granify monitors online shopper behavior in real-time by analyzing many attributes to show personalized incentives and pricing. The machine learning engine also predicts which in-session prospects you may lose and then suggest ways to convert them.
- Personali creates personalized incentives to increase sales. The machine learning algorithms developed by data scientists and behavioral economists perform precision targeting with intelligent incentives.
- Wacul-AI provides user-friendly proposals and solutions to enhance the entire website. It gives simple pieces of advice with their predicted future outcomes by analyzing data.
To thrive in the e-commerce industry, retailers should be as agile as possible, and machine learning is the way to do so. Machine learning is a necessary investment for e-commerce players.
Leave a reply or comment below